Biography and research interest
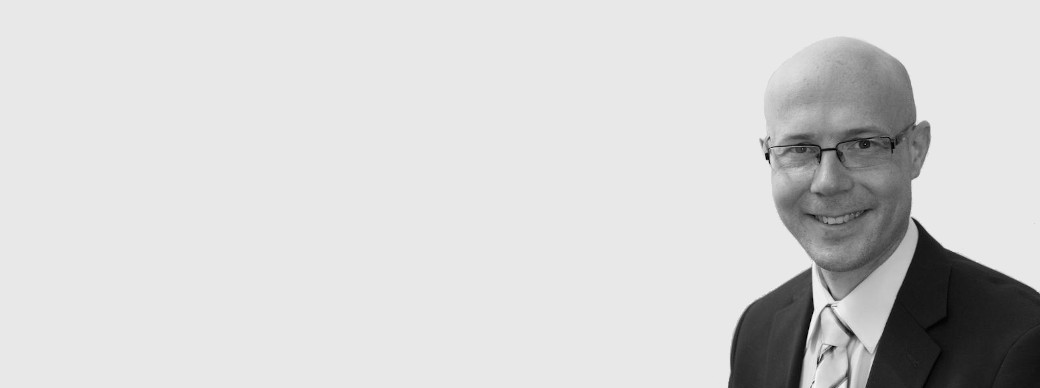
A short biography
I performed my doctoral work at Texas A&M University in the Department of Mathematics. The focus area of my dissertation was in numerical analysis and scientific computing with an emphasis on finite element methods applied to computational fluid dynamics. After completing a Ph.D. I held a software development position at the Joint Institute for Computational Sciences at Oak Ridge National Laboratory. I returned to academics in 2015 when I accepted a Pfeiffer postdoctoral fellowship at Rice University. There, I designed and analyzed DG methods for poroelasticity. I applied these methods to study intestinal neuropathology and experimental fluid resuscitation alongside clinical researchers from UT Health Science Center. From 2017 to 2019, I held a postdoctoral fellow position at Simula Research Laboratory in Oslo, Norway. At Simula, my research focused on mixed finite element methods, for applications to patient-specific human brain modeling, used in the solution of the equations of generalized poroelasticity. From 2019-2022, I held a postdoctoral research appointment, within the Mathematical Institute, at Oxford University where I designed, analyzed and simulated mathematical models for neurodegenerative diseases in the human brain. I also co-advised several Oxford D.Phil. students and applied my mathematical models to study Alzheimer's disease alongside biomedical engineers, theoretical chemists, neurologists and clinical researchers from the United States, Norway and the United Kingdom. In the Fall of 2022, I had the good fortune to join the faculty of Texas Tech University in Lubbock, Texas where I currently hold the position of Assistant Professor in the Department of Mathematics.
Research interest
My research program develops applied and computational mathematical methods and data-driven models to study the brain, biological processes on complex networks and neuropathologies such as Alzheimer's disease. To accomplish this goal, my work combines theory from ordinary and partial differential equations with numerical analysis and high-performance computing, alongside new initiatives in data-driven inference and machine learning.